What Can We Learn from Modeling a Pandemic?
Individual-based modeling offers untapped opportunities for policymakers and researchers
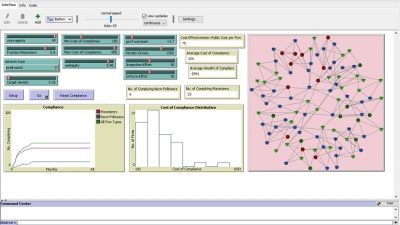
With the emergence of the corona virus, modeling – the science of representing processes and systems for purpose of analysis—has been at the center of debate how to respond to this public health crisis. Experts and non-experts alike follow the latest modeling predictions, and federal and state public health policies are grounded, at least in part, on those constantly shifting predictions. That got me thinking—what can legal scholars and environmental regulators learn from the use of such epidemiological models in the public health context? The answer is a lot.
First, let’s clear away some of the brush. Epidemiologists use a variety of different types of models to predict the course of epidemics and pandemics. Here are the three main types:
• Empirical statistical models are fitted to past data. For example, the Institute for Health Metrics and Evaluation (IHME) model, which the White House Coronavirus Task Force relies upon heavily, extrapolates US mortality growth curves from reported worldwide deaths. The IHME model, which has come under fire from various quarters, does not focus on the dynamics of virus transmission or epidemiology, or differences across countries that may influence individuals’ responses to public health policies.
• “SEIR” models break the population into four categories: susceptible (S), exposed (E), infected (I) and recovered (R). SEIR models use differential equations to chart the relative growth (and decline) in each category over time. These models do not capture the system-wide impacts of individual decisions and interactions with the population and within institutions like hospitals or governments, including impacts on the nature and efficacy of social distancing and quarantine policies.
• Individual-based modeling (IBM), also known as agent-based modeling, is the newest on the scene. IBM is most useful in dealing with complex adaptive systems in which interconnected entities are organized into a collective whole that uses information, generates (sometimes unpredictable) patterns and behaviors, and learns or evolves over time. Think here of a society grappling with a pandemic—that’s what researchers at the Imperial College London did in developing their IBM for influenza and now for Covid-19. An IBM models from the bottom up, using software to simulate a population of virtual, heterogeneous agents having unique attributes, preferences and decision-making rules. (The agents’ decision rules, preferences and other attributes can be based on theory or, where available, on empirical data about an actual population of interest.) The agents, which could include individuals and institutions such as hospitals, businesses or governments, interact with one another and their environment, and can adapt to changes in that environment including the implementation of various public health interventions. The model tracks behavior of the agents and the associated outcomes, in this case, public health metrics such as mortality and health care demand.
There’s a reason IBM models have emerged as such a powerful tool guiding our decision-makers during the corona virus pandemic. Unlike many conventional forms of modeling for social and economic systems, IBM can incorporate social norms and heuristics into decision-making and take into account the effects of learning and adaptation. These features are critical in understanding the dynamics of complex systems.
Unsurprisingly, researchers in a range of disciplines—ecology, sociology, political science, public policy, engineering and even economics—increasingly use IBM to study the complex social, technical and natural systems of interest to them. Although its use by environmental policy makers has been largely limited to a just a few settings, IBM could significantly improve policymakers’ capacity to design policies that maximize environmental outcomes. IBM allows policymakers and researchers to take alternative polices for a test drive, running hundreds or thousands of simulations to understand how changes in the policies or assumptions about individual behavior and their environment could affect outcomes. For example, IBM has been used to model the impact of various incentive programs for adoption of “green” technologies such as electric vehicles, rooftop solar and energy efficient appliances. Another project evaluated the outcomes flowing from implementation of the EU’s REACH chemicals regulation.
IBM simulation can also be an effective teaching tool, allowing students to explore the nuances of the theory underlying various types of regulation. In my seminar on the regulation of business firms, we use an IBM model of a virtual industry sector to play with enforcement policies and challenge assumptions regarding the factors that drive business behavior. Students work in groups to develop and test hypotheses regarding individual behavior and system outcomes. The model provides an engaging look under the hood of compliance and enforcement theories, linking them to on-the-ground outcomes in a compelling way.
Reader Comments
2 Replies to “What Can We Learn from Modeling a Pandemic?”
Comments are closed.
Great summary of empirical models compared to IBMs. If computers had been invented before calculus, empirical models would never have been needed. In fact, calculus would not have been needed either.
Well said…